Ivana Dusparic & Pieter Barnard
Explainable Machine Learning for Optimization of Resource Use in Large-scale Heterogeneous Infrastructures
The use of artificial intelligence (AI), and in particular reinforcement learning (RL) and deep neural networks (DNN), is being extensively investigated in a range of large-scale autonomous systems. This talk will focus on some of the new AI techniques we are developing for optimization of resource use in urban environments, for example, intelligent urban traffic networks, smart grid, sensor networks, and wireless communication networks. These city-scale infrastructures share properties with many other large-scale autonomous systems, i.e., are characterized by distributed control, heterogeneity, presence of multiple and often conflicting goals, reliance on diverse sources of information, and the need for continuous adaptation. We discuss the issues in applying RL in such environments and present the techniques we have developed to enable multi-agent multi objective optimization, adaptation in non-stationary environments, and continuous knowledge transfer via parallel transfer learning. We particularly focus on the need for explainability of AI decision-making processes, and present two of our recent explainability techniques - in the first work, we present a novel technique to detect causal confusion during the decision-making process of an RL agent, while our second work discusses the evolving role that explanations have within the ML pipeline, by not only engendering greater trust in the ML solution but also allowing its overall performance to be enhanced. We conclude by discussing further challenges in enabling RL and DNN deployments in autonomous systems.
back to overview
Watch Recording
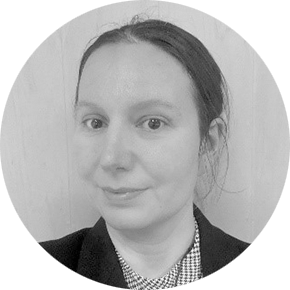
Biography
Dr. Ivana Dusparic is an Ussher Assistant Professor and a recently elected Fellow of Trinity College Dublin. Her research expertise is the development of new AI algorithms for optimization in large-scale infrastructures. She has authored over 80 peer-reviewed articles in the areas of reinforcement learning agents, multi-agent systems, intelligent mobility, and future communication networks. She is a co-director of SFI Centre for Research Training in AI, Principal Investigator of the SFI-NSFC Smart Networking in the Era of AI collaboration between Trinity College Dublin and Tsinghua University, a co-lead of ClearWay, an newly funded project on Advancing Deep Reinforcement Learning and Swarm Intelligence for intelligent mobility, and a funded investigator in CONNECT – SFI Research Centre for Future Networks and Communications.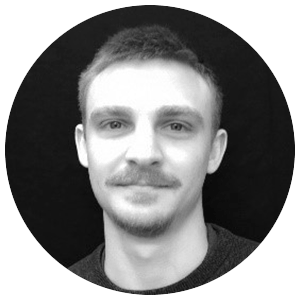