Freddy Lecue
Uncovering the Semantics of Deep Neural Networks with Knowledge Graphs
We are currently seeing is a surge of innovation and uptake focused on machine learning, and more specifically deep learning — which is most successful in low-level pattern recognition tasks from many digitalized content such as image, video, speech or text. Today’s machine learning systems are achieving impressive results, having demonstrated wide applicability with real-world impact in many contexts. Latest results are GitHub Copilot powered by Open AI Codex and its customized version of GPT-3, or Gato from DeepMind, which works as a multi-modal, multi-task, multi-embodiment generalist policy, able to generalize beyond expectation. Powered by deep neural networks, the inner semantics of their mechanics remains largely opaque an open to lots of unanswered questions, making the engineering of any new architecture very brittle. This presentation will present some results and directions towards uncovering the semantics of deep neural networks using knowledge graphs to scale the engineering of deep neural networks.
back to overview
Watch Recording
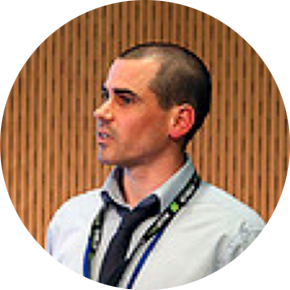