MingMing Liu
On the Design of Privacy-Aware Smart Mobility Systems: From Decentralised Optimisation to Federated Learning
Privacy-aware Smart Connected Mobility Systems: From Decentralised Optimisation to Federated Learning
In the rapidly evolving landscape of intelligent transportation systems, the design and implementation of privacy-aware smart mobility systems have become critical. As these systems become increasingly connected, the ability to integrate advanced privacy-preserving mechanisms becomes not only beneficial but essential. This enables the development of smarter, more efficient transportation solutions that users can trust with their data.
In this presentation, I shall focus on two innovative approaches in two advanced smart mobility use cases. First, I will delve into the use of decentralised optimisations for the design of privacy-preserving speed advisory systems. The main goal of this application is to recommend a consensus speed for a group of vehicles to minimise emissions by solving a constrained optimisation problem without revealing cost functions of individual vehicles. Following that, I will introduce how Multi-party Computation (MPC) methods can be applied to the system design to significantly accelerate algorithm convergence for real-time decision-making whilst maintaining privacy for users.
Next, I will discuss Federated Learning (FL) for improving energy consumption modelling in connected Battery Electric Vehicles (BEVs). By employing strategies such as FedAvg and FedPer, FL can significantly improve predictive accuracy while protecting user privacy. Leveraging local model updates instead of direct data sharing enables more efficient route planning and energy management of BEVs, alleviating users' range anxiety. Finally, I will demonstrate the implementation of this application in a real-world edge-cloud computing framework for greater societal impact.
Attendees will gain insights into effectively using these advanced technologies to enhance the efficiency and privacy of these systems, paving the way for future developments in related fields.
In the rapidly evolving landscape of intelligent transportation systems, the design and implementation of privacy-aware smart mobility systems have become critical. As these systems become increasingly connected, the ability to integrate advanced privacy-preserving mechanisms becomes not only beneficial but essential. This enables the development of smarter, more efficient transportation solutions that users can trust with their data.
In this presentation, I shall focus on two innovative approaches in two advanced smart mobility use cases. First, I will delve into the use of decentralised optimisations for the design of privacy-preserving speed advisory systems. The main goal of this application is to recommend a consensus speed for a group of vehicles to minimise emissions by solving a constrained optimisation problem without revealing cost functions of individual vehicles. Following that, I will introduce how Multi-party Computation (MPC) methods can be applied to the system design to significantly accelerate algorithm convergence for real-time decision-making whilst maintaining privacy for users.
Next, I will discuss Federated Learning (FL) for improving energy consumption modelling in connected Battery Electric Vehicles (BEVs). By employing strategies such as FedAvg and FedPer, FL can significantly improve predictive accuracy while protecting user privacy. Leveraging local model updates instead of direct data sharing enables more efficient route planning and energy management of BEVs, alleviating users' range anxiety. Finally, I will demonstrate the implementation of this application in a real-world edge-cloud computing framework for greater societal impact.
Attendees will gain insights into effectively using these advanced technologies to enhance the efficiency and privacy of these systems, paving the way for future developments in related fields.
back to overview
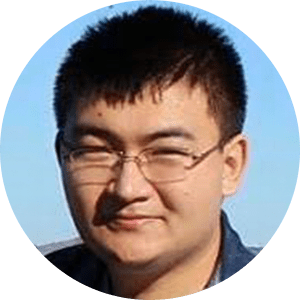